Gripping and manipulating items of diverse shapes and sizes has long been one of the biggest challenges facing industrial robotics. The difficulty is perhaps best summed up by the Polanyi Paradox, which states that we "know more than we can tell." In essence, while it may be easy to teach machines to exhibit a high level of performance on tasks that require abstract reasoning such as running computations, it is substantially harder to grant them the sensory-motor skills of even a small child in all but the most standardized and predictable environments.
However, with the need for flexible picking to accommodate reduced changeover time for more varied product runs on the rise, industry is pursuing new solutions to the problem.
A new collaboration project between Festo and the Karlsruhe Institute of Technology (KIT) called FLAIRPOP (Federated Learning for Robot Picking), seeks to use training data from multiple stations, plants, or even companies to more effectively drive deep learning algorithms with the goal of helping picking robots to become more adaptable. The approach is similar to that used by cloud-driven machine learning algorithms, which leverage larger amounts of data than individual end-users have access to.
According to Jonathan Auberle from the Institute of Material Handling and Logistics at KIT, the process works by allowing autonomous robots at multiple different picking stations to grip and transfer items of various shapes and sizes. This data is then aggregated and shared, allowing other robots to more effectively manipulate objects they have not yet encountered.
At that, the technique isn’t entirely new. It’s already been applied with substantial success in the medical sector where it is used for image analysis. Moreover, because the protection of patient data is a high priority in the medical field, it is well-equipped by design to protect intellectual property, even in situations where data from numerous companies is synthesized, according to Jan Seyler, head of advanced development for analytics and control at Festo.
“In the FLAIROP research project, we are developing new ways for robots to learn from each other without sharing sensitive data and company secrets. This brings two major benefits: 1) we protect our customers' data and 2) we gain speed because the robots can take over many tasks more quickly. In this way, the collaborative robots can, for example, support production workers with repetitive, heavy, and tiring tasks,” Seyler said.
KIT will contribute its expertise in robotics, deep learning, and data security to the project, while Festo will be responsible for pilot deployments in a real-world environment. In addition, the pilot deployments will use start-up DarwinAI’s Explainable AI (XAI) platform, which is capable of clearly illustrating for human operators why artificial intelligence (AI) algorithms behave the way they do.
“We hope that our XAI technology will enable high-value, human-in-the-loop processes for this exciting project, which represents an important facet of our offering alongside our novel approach to federated learning. Having our roots in academic research, we are enthusiastic about this collaboration and the industrial benefits of our new approach for a range of manufacturing customers,” said Sheldon Fernandez, CEO at DarwinAI.
Companies in this Article
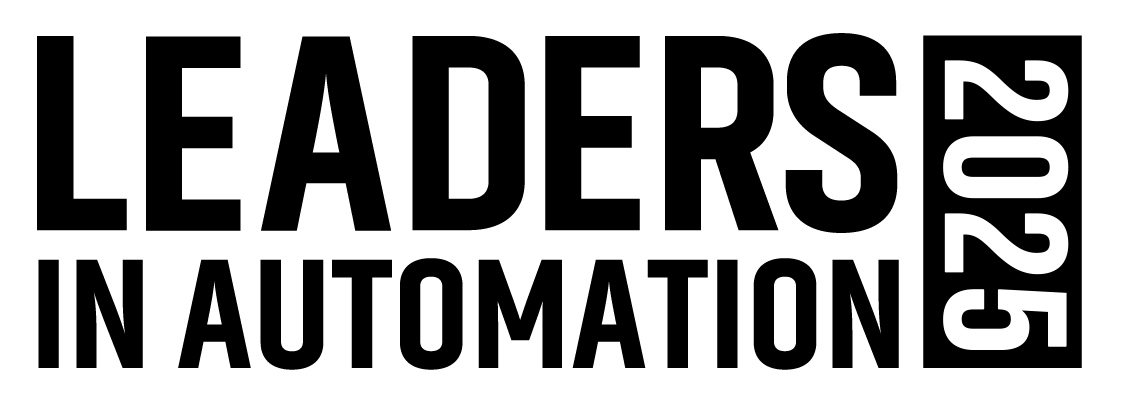
Leaders relevant to this article: