As the era of Industry 4.0, with its promises of predictive analytics, integrated business planning, and increased operational efficiencies continues to heat up, Big Data is a topic on everyone’s mind. Yet, with so much discussion of the potential value to be leveraged from the growing quantities of data being generated by all manner of sensors and devices, less attention is being paid to the all-too-necessary precursor of effective analytics—data quality.
In this domain, the age-old maxim “garbage in, garbage out” still reigns supreme. Even the most advanced machine learning algorithms are useless when fed poor quality data.
“Data quality is everything,” says Tom Redman, president at Data Quality Solutions. “The first thing is that if you’re using existing data to train a model and you don’t do a really good job cleaning it up, you’re going to get a bad model. Even if the model [you construct] is good, if you put bad data into it, you’re just going to get a bad result. If you stack these things up, it’s like a cascade, and the problem will quickly get out of control.”
So, how does one define what is or isn’t quality data? This is a challenging question because much of the answer depends on the particular problem you’re looking to solve. Generally speaking, the quality of data can be measured in accordance with four primary dimensions: accuracy, consistency, completeness, and timeliness.
If values that have been gathered from across a network have accuracy, they properly reflect the information that was produced by each device. For example, if several devices within a single space are all reporting the ambient temperature in that area, data analysts should expect those values to either be the same or within a reasonable deviance from one another. Consistency is similar. When data is consistent, it means that multiple events reported under similar conditions do not exhibit irreconcilable variances. In contrast, completeness is attained when there are no substantial gaps in a time-series of reported events or captured values from sensors. Finally, if data possesses timeliness, it means that it has been able to pass from its initial point of creation through various communication protocols and levels of integration into a data management platform where it can be synchronized with data from other sources quickly enough to effectively be acted on.
While such a whirlwind of metrics and criteria may seem convoluted, Redman says that it can all be boiled down into two simple axioms. Data must be right, meaning that it’s accurate, consistent, and complete. It must also be the right data, which entails not only meeting technical standards of quality, but being unbiased and pertaining to the particular range of inputs for which one aims to develop a predictive model. Poorly calibrated equipment may be responsible for shortcomings in the former, but the latter is especially important because it calls on the insight and creativity of human analysts and their ability to communicate their needs to operational technicians who create data further upstream.
Ensuring data quality from the outset
Redman’s approach to ensuring data quality differs from some others in that, while he acknowledges technology is important, he believes that it is first and foremost a management concern. In his view, when communication between data creators and data users is made clearer, it becomes exponentially easier to not only collect the data that is right, but the right data.
“One thing that you’ll notice is that no one ever really creates bad data if they’re going to use it themselves, but a lot of data is created the first time in one part of an organization and not used until somewhere downstream in another part of it. People go along blithely creating the data, and then the people who have to use it say, ‘Oh, this is no good,’ and so they have to clean it,” he says. “It never occurs to them that maybe they should figure out who’s creating the data and go down there and have a little chat about their requirements. The goal of data quality should be to get out of the cleaning business altogether.”
In other words, a conscious decision needs to be made to develop methods of communication between the various members of an organization whereby the requirements of all data being generated can be clearly delineated. Redman sees this as management’s responsibility to impose, and, if necessary, to provide training for as well.
And while Redman stresses that kinks in the communication pipeline should be fully sorted out before an organization rushes to more sophisticated technological approaches, once a strong workflow has been put in place by management, investing in the right hardware and software is also important.
Increasing data cleaning efficiencyGiven the strenuousness of a data janitor’s work, Redman’s stance isn’t surprising. According to Anil Datoo, vice president of data management at Emerson, around 70% of all data integration activities are spent validating, structuring, organizing, and cleaning data, a statistic that was echoed in an article on Big Data in The New York Times in 2014. With so much time being committed to the task of data cleaning and very little headway having been made to reduce it over the past half-decade, working to ensure that more data is in tip-top shape from its inception isn’t a bad strategy.
However, even if such measures can ultimately ease the burden of handling data, the sheer volume being created renders it nearly impossible that cleaning can be bypassed altogether, says Michael Risse, vice president and chief marketing officer at Seeq. Moreover, it’s often difficult to know precisely what data will be needed until a new problem has emerged. As a result, software tools that enhance the efficiency of sorting and cleaning large volumes of data can be an invaluable tool, even in the most organized managerial regimes.
"A critical part of this conversation is that data must be right for your particular analytic. One thing that's vital is making sure the original data is stored in its raw form. If it's summarized—because someone assumed they knew what I wanted to do with it, or it is already somehow altered or cleansed based on someone else's expectations—it might in fact be ruined," Risse says. "I might be looking for exactly the anomalies or outliers which someone else thinks should be removed. One of the big challenges of all of this is that we often don't know what we're going to need until we need it."
From Risse’s perspective, while improving data quality from the outset of an operation might be useful in some contexts, in others, over-sanitizing what’s available could actually create further problems, even under the strictest forms of guidance. That’s why Seeq’s software is designed to help users parse large quantities of data more rapidly so that it doesn’t need to be summarized or curtailed earlier on in the pipeline.
A use-case can help illustrate the utility of Seeq’s software. Take, for example, an industry that employs batch production, like pharmaceuticals or food and beverage. Often, if a quality issue emerges, manufacturers will simply dump an entire batch and start production over rather than try to identify the source of the problem because the analytics necessary to do so are so time-consuming that, by the time they have been conducted, the batch will be completed anyway. Using Seeq’s software, the process can be accelerated so that decisions can be made quickly enough to have an impact on the outcome.
“In one example at a refinery we worked with, it was taking them two weeks to get insights on their daily production,” Risse says. “Now, they can turn that decision around in an hour and increase production every year with the same plant, the same assets, and the same people.”Managing expectations for digital transformation initiatives
For those looking to take the plunge in pursuit of becoming a more data-savvy organization, Redman, Datoo, and Risse all have valuable advice to offer.
First and foremost, Datoo recommends beginning the transition with a small, targeted project, rather than diving in all at once.
“Our main recommendation is just to start and develop a small use-case. It doesn’t have to be cost prohibitive because there’s a lot of opportunity within operating environments; so if you can just target something that resonates operationally, would have a good return on investment, and can get the attention of operational stakeholders, you’re set,” he says. “Continue to measure success along the way, be flexible, and expect to make iterative changes. There’s no simple answer to these problems, so it’s important to allow for that.”
Similarly, Redman urges adopters to pick a specific problem and stick to it. Too often, he says, organizations make the mistake of hiring data scientists, giving them access to all of the organization’s information, and waiting to see what they come up with. In Redman’s opinion, such undisciplined approaches are doomed to failure.
Managing expectations is important too. While improving data quality at the outset is possible with a managerial focus and the tools to improve data cleaning efficiency, organizations shouldn’t expect their digital transformations to reinvent their business overnight, Redman cautions. At the same time, Seeq’s use cases show that, when used properly, data insights can, with time, unlock the key to truly transformative results.
Most importantly, data quality should remain at the core of all digital transformation initiatives. Just as a chef is often only as good as the ingredients provided, data scientists are likewise limited by bad data.
In addition, the two-pronged approach of eliminating the root cause of poor-quality data through management innovations and parsing data more effectively through the use of advanced software points to the far-reaching effects of industry’s digital revolution. Far from being the sole province of a few computer analysts, Big Data touches every segment of industry, from operations to administration. And while adapting to this new paradigm isn't without its challenges, businesses hoping to thrive in this era of uncertainty must be prepared to do so.
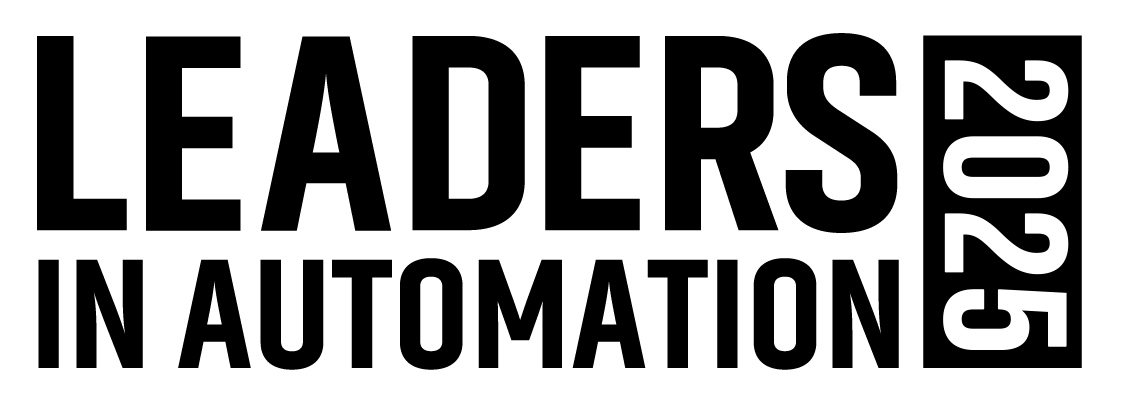
Leaders relevant to this article: